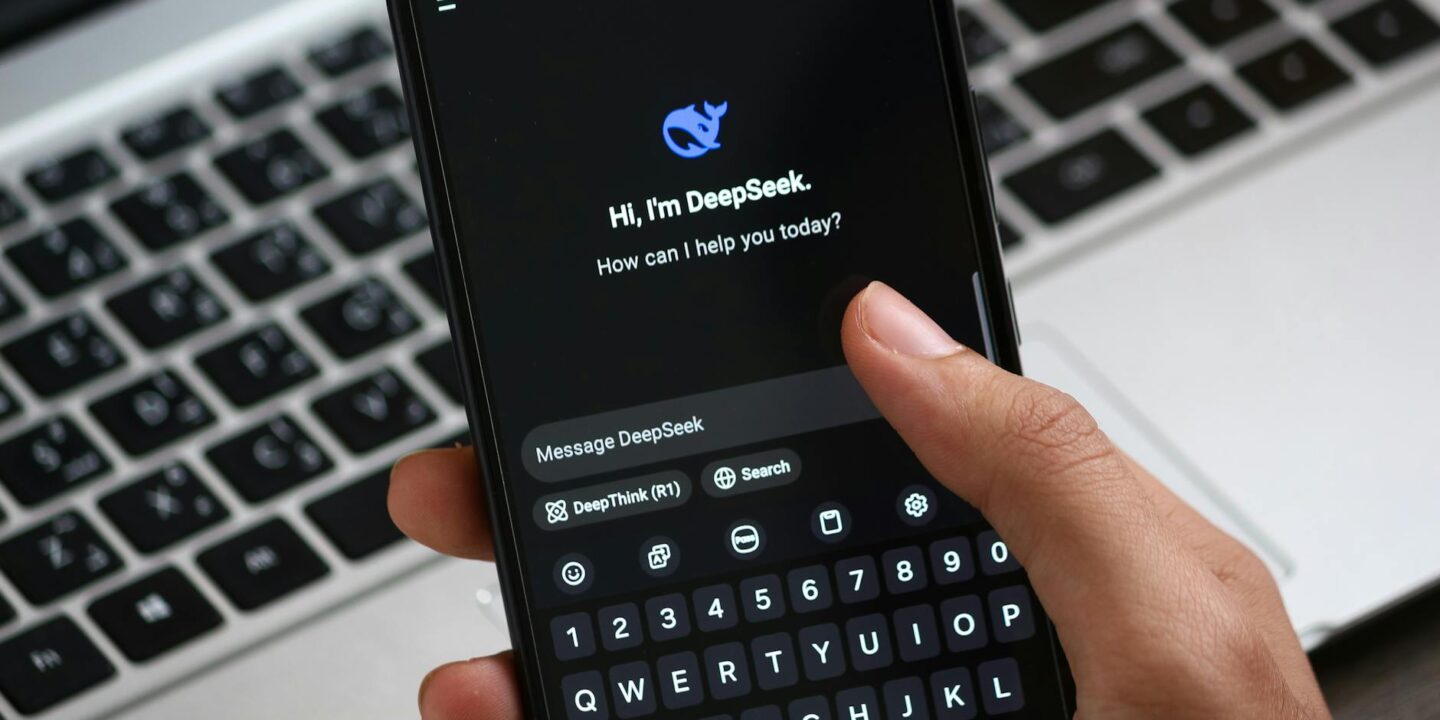
Reliability of AI Detectors
When considering the reliability of AI detectors, it’s essential to evaluate their accuracy and the limitations they face. These tools, often referred to as AI busted solutions, are designed to identify text that may have been generated by AI, such as ChatGPT, and are increasingly used in various fields, including education and marketing.
Accuracy of AI Detection Tools
The accuracy of AI detection tools can vary significantly. The highest accuracy reported for premium AI detectors is around 84%, while the best free tools achieve about 68% accuracy. While these figures provide a useful indication of the likelihood that a text was AI-generated, they are not definitive proof on their own (Scribbr).
Tool Type | Accuracy (%) |
---|---|
Premium Tool | 84 |
Best Free Tool | 68 |
Despite these numbers, the accuracy of AI detectors remains a concern. Instances of false positives and failures to detect AI-generated content highlight the limitations of the underlying algorithms and the training data used.
Limitations of AI Detectors
AI detectors face several limitations that impact their reliability. One significant issue is the reliance on biased or insufficient training data, which can lead to partiality in predictions and unfair outcomes (AI Contentfy). This bias can result in discriminatory results, making it crucial to use diverse and representative training data to enhance the accuracy and fairness of these tools.
Another challenge is the balance between overfitting and underfitting. Overfitting occurs when a model is too complex and learns noise from the training data, while underfitting happens when a model is too simple to capture the underlying patterns. Techniques like regularization and cross-validation are essential to mitigate these issues and improve the overall accuracy of AI detectors.
For more insights into the errors that AI detectors can make, check out our article on what are the errors in ai detectors?. If you’re curious about whether AI busted tools can detect AI in academic papers, visit can ai be detected in papers?.
Enhancing AI Detection Accuracy
To understand how reliable AI detectors are, it’s essential to explore the factors that affect their accuracy and the strategies that can improve their reliability.
Factors Affecting Detection Accuracy
Several elements can influence the performance of AI detection tools. Here are some key factors:
Factor | Description |
---|---|
Perplexity | This measures how predictable a text is. Low perplexity can indicate AI-generated content. |
Burstiness | This refers to the variation in sentence length and complexity. Low burstiness may also suggest AI authorship. |
Editing | If AI-generated text is edited to appear more human-like, detectors may struggle to identify it. |
Prompting | AI outputs that are specifically prompted to be less predictable can confuse detection tools. |
Data Evolution | AI detectors must adapt to new writing styles and trends to maintain accuracy. |
AI detectors are based on language models similar to those used in AI writing tools. They primarily look for perplexity and burstiness in a text to determine its likelihood of being AI-generated (Scribbr).
Strategies for Improving Reliability
Improving the reliability of AI detection tools involves several strategies:
- Regular Model Updates: Continuously adapting AI models to evolving data and patterns is crucial. This ensures that the detectors can accurately analyze new writing styles and trends (AI Contentfy).
- Utilizing Multiple Metrics: Employing various metrics such as precision, recall, and F1 score can provide a comprehensive assessment of detector performance. The F1 score balances precision and recall, offering a better gauge of overall accuracy (AI Contentfy).
- User Feedback: Incorporating user feedback can help refine detection algorithms. By understanding how users interact with the tool, developers can make necessary adjustments to improve accuracy.
- Testing Against Diverse Datasets: Regularly testing AI detectors against a wide range of datasets can help identify weaknesses and areas for improvement. This practice ensures that the tools remain effective across different writing styles and contexts.
- Combining Tools: Using multiple detection tools in conjunction can enhance reliability. Each tool may have unique strengths, and combining their outputs can lead to more accurate assessments.
By understanding the factors that affect detection accuracy and implementing these strategies, you can enhance the reliability of AI detectors. For more insights on the limitations of these tools, check out our article on what are the errors in ai detectors?. If you’re curious about the effectiveness of AI detection in academic settings, visit can ai be detected in papers?.