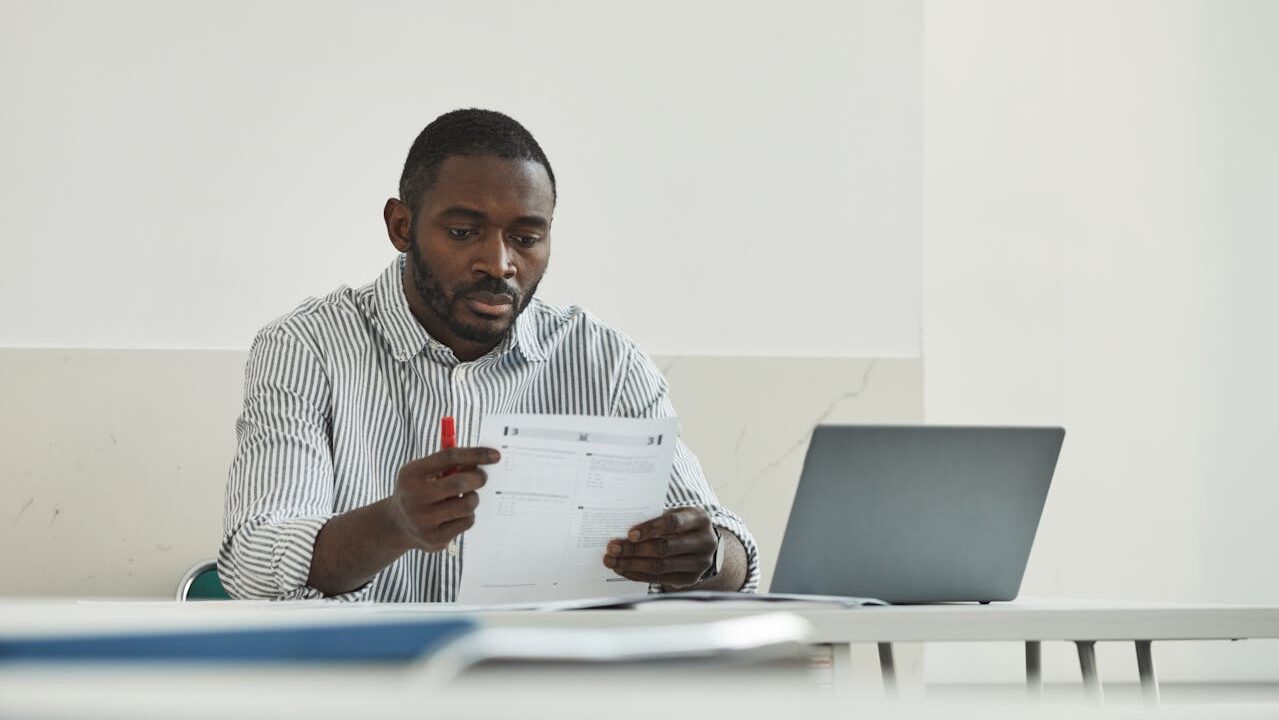
Understanding AI Detection
As you explore the world of AI detection, it’s essential to understand the factors that contribute to errors in these systems. The emergence of AI busted cases reveals the complexities behind these tools and helps highlight where improvements are needed. This knowledge can help you navigate the complexities of AI and its implications for your work.
Sources of AI Errors
AI detection systems can make mistakes for several reasons. Errors often stem from probabilistic pattern matching, hallucinations, and other complexities inherent in AI systems. These issues can lead to incorrect assessments or outputs that do not align with reality (IBM).
Here are some common sources of errors in AI detection:
Source of Error | Description |
---|---|
Probabilistic Pattern Matching | AI systems rely on patterns in data, which can lead to inaccuracies if the patterns are not representative. |
Hallucinations | AI may generate outputs that are not based on real data, leading to misleading results. |
Data Quality | Poor quality or biased data can result in flawed AI outputs. |
Understanding these sources can help you critically evaluate the reliability of AI detection tools. By recognizing AI busted scenarios, you can better understand when and why certain errors occur.
Impact of Bias in AI
Bias in AI is a significant concern that can affect the accuracy and fairness of AI detection systems. Bias can occur at various stages of the AI pipeline, with one of the primary sources being data collection. If the data used to train an AI algorithm is not diverse or representative, biased outputs may result (Chapman University).
AI systems can internalize implicit biases from their training data. For example, if a model learns from biased language or imagery, it may unknowingly generate prejudiced or stereotypical outputs (Chapman University). This can have real-world consequences, such as making people less willing to acknowledge the problem of biased outputs. These issues are not limited to image generators but also extend to text generators like ChatGPT (MIT Sloan Teaching & Learning Technologies).
Addressing bias in AI requires a multifaceted approach, including proactively identifying and mitigating biases to create AI systems that contribute to a more equitable and just society. For more insights on the challenges of AI detection, check out our article on what are the problems with ai detection?.
Understanding these factors will help you navigate the complexities of AI detection and its implications for your work. If you have questions about specific tools, you might wonder, why is an ai detector saying my writing is ai? or can using grammarly be flagged as ai?.
Reliability of AI Detection
When considering the question, can AI detection be wrong?, it’s essential to understand the challenges and limitations that come with these tools. While AI detectors are becoming more popular, they are not infallible.
Challenges in AI Detection
AI detectors are designed to identify text that has been partially or entirely generated by AI tools like ChatGPT. They are commonly used by educators to evaluate student writing and by moderators to filter out fake reviews and spam content. However, these tools face several challenges:
- Complexity of Language: AI detectors analyze text based on perplexity and burstiness. These metrics assess how predictable or varied the text is. If an AI-generated text is prompted to be less predictable or is edited after generation, the detector may struggle to identify it accurately.
- Variability in Performance: The reliability of AI detectors can vary significantly. The highest accuracy reported is around 84% for premium tools, while the best free tools achieve about 68% accuracy. This means that even the most reliable detectors can produce false positives or negatives.
- Evolving AI Capabilities: As AI writing tools improve, they become better at mimicking human writing styles. This evolution makes it increasingly difficult for detectors to differentiate between human and AI-generated text.
Detector Type | Accuracy Rate |
---|---|
Premium Tool | 84% |
Best Free Tool | 68% |
Limitations of AI Detectors
Despite their growing use, AI detectors have notable limitations:
- Not Definitive Evidence: AI detection tools provide an indication of the likelihood that a text was AI-generated, but they do not offer definitive proof. This means that a high score on an AI detector does not guarantee that the text is AI-generated.
- Plagiarism Concerns: AI-generated text may sometimes include sentences that are directly copied from existing sources. This can lead to AI-generated content being flagged as plagiarism by traditional plagiarism checkers, complicating the assessment process further.
- Contextual Misinterpretation: AI detectors may misinterpret the context of certain phrases or styles, leading to incorrect classifications. This can be particularly problematic in creative writing or when using specific jargon.
For more insights into the challenges faced by AI detection tools, check out our article on what are the problems with AI detection?. If you’re curious about why an AI detector might flag your writing as AI-generated, visit why is an AI detector saying my writing is AI?.