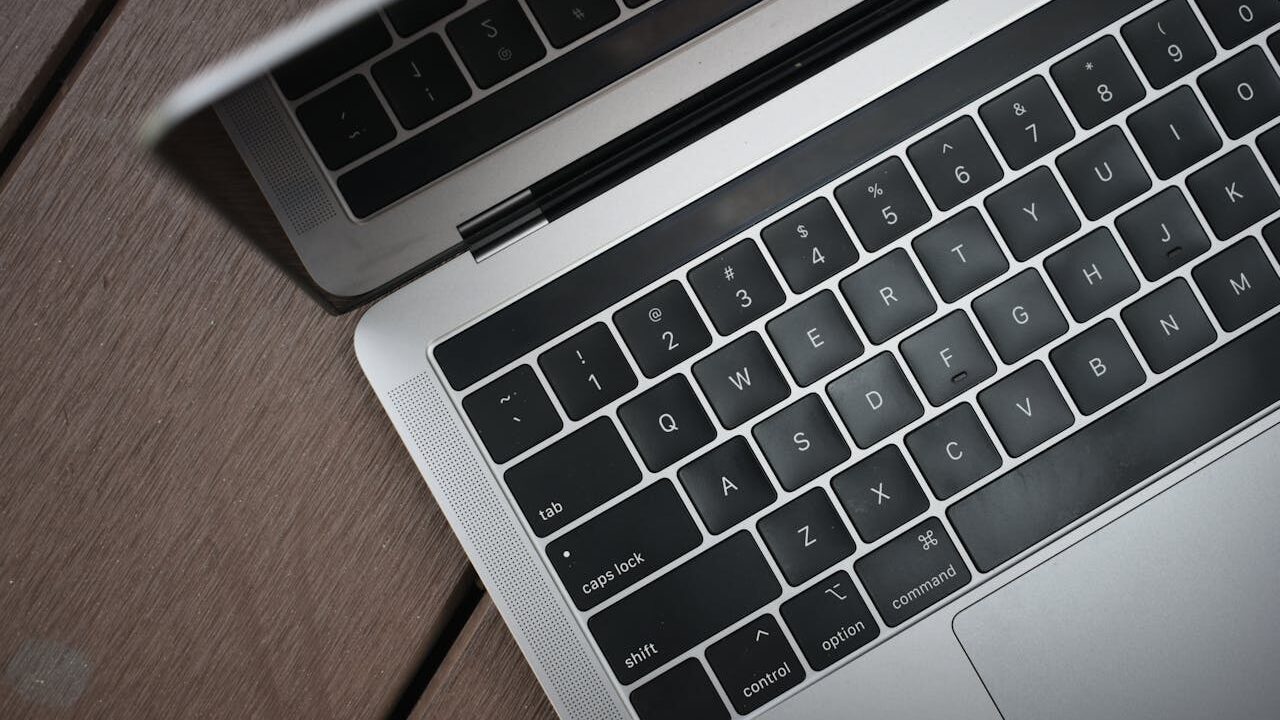
Understanding AI Detectors
AI detectors play a critical role in distinguishing between human and machine-generated writing. Understanding their classification accuracy and how writing styles affect their performance can help you navigate potential pitfalls in AI detection technology.
Classification Accuracy of AI Detectors
The accuracy of AI detectors can vary significantly based on the writing style of the text being analyzed. For instance, research highlights that AI detectors were able to accurately classify essays written by U.S.-born eighth-graders but struggled with essays authored by non-native English speakers. Over half (61.22%) of the TOEFL essays from non-native students were misclassified as AI-generated, demonstrating a potential bias in these tools.
Here’s a summary of the classification accuracy based on writing backgrounds:
Writing Background | AI-Generated Classification Rate |
---|---|
Non-Native English Writers | 61.22% |
U.S.-Born Eighth-Graders | Near-perfect |
Total Essays Analyzed | 91 |
Essays Flagged as AI | 97% (at least one detector) |
This information indicates that while AI detectors may be reliable in some scenarios, they can misclassify certain types of writing, particularly if the writers lack proficiency in English.
Impact of Writing Styles on Detection
Writing style is a crucial factor that affects the performance of AI detectors. Non-native writers often exhibit lower scores on metrics like lexical richness, diversity, and syntactic complexity. These differences can lead to challenges in detection and may result in such writing being flagged erroneously as AI-generated (Stanford HAI).
The bias toward certain writing styles can impact the overall accuracy of the detectors. If the training dataset is not adequately diverse—representing various writing forms—the AI may struggle with different styles. For example, some detectors may over-represent more complex writing styles due to the dataset they were trained on, inadvertently leading to misclassifications of simpler or less intricate texts (CSUDH University Library).
When considering which tools to use for detecting AI-generated content, it’s essential to factor in these limitations. You can explore more about various AI detection tools and how they stack up against established platforms like Turnitin in our article on which ai detector is closest to turnitin?. Understanding these dynamics will better equip you for navigating the complexities of AI detection technology.
Reliability Challenges of AI Detectors
AI detectors, while useful in identifying AI-generated content, face several reliability challenges that question their effectiveness and fairness. Below, you’ll find an overview of these challenges, focusing on prompt engineering and ethical implications.
Prompt Engineering and Detection
Prompt engineering refers to the technique used to manipulate AI responses, allowing for more complex language that can evade current detection models. You might wonder how this affects the reliability of AI detectors. According to research, AI detectors can be easily subverted by “prompt engineering,” where generative AI is asked to “rewrite” essays with sophisticated language, making it harder for detectors to identify the content as AI-generated (Stanford HAI). This poses a significant challenge to their accuracy, as these models weren’t designed to account for such modifications.
AI Detection Challenge | Description |
---|---|
Prompt Engineering | Techniques to manipulate language to evade detection. |
Detection Accuracy | Reduced effectiveness in identifying rephrased or sophisticated content. |
Model Limitations | Current models are not fully equipped to handle advanced language manipulation. |
In these situations, the “AI busted” phenomenon becomes not just a technical issue but an ethical one, underscoring the importance of fair and transparent AI systems.
Ethical Concerns and Implications
The challenges that AI detectors face also lead to serious ethical concerns regarding their deployment. The unreliability and vulnerability of these detectors can result in unfair accusations or penalties, particularly for foreign-born students and workers, who may have different writing styles that are misclassified. Consideration of bias is critical, especially if the training samples used to develop these models are not diverse or representative of various writing styles.
Here’s a quick overview of the ethical implications:
Ethical Concern | Description |
---|---|
Fairness | Misclassification could lead to penalties for non-native speakers. |
Bias in Training Data | Lack of representation can distort detection accuracy. |
Need for Caution | Important to rigorously evaluate AI detector reliability before usage. |
When exploring which AI detector is closest to Turnitin?, it’s essential to consider these factors along with the model’s accuracy. This will provide a more comprehensive understanding of the challenges and limitations inherent in AI detection technologies. Further insights can also be gained from discussions around whether universities can detect ChatGPT if you paraphrase, as implications stretch beyond just the technology.