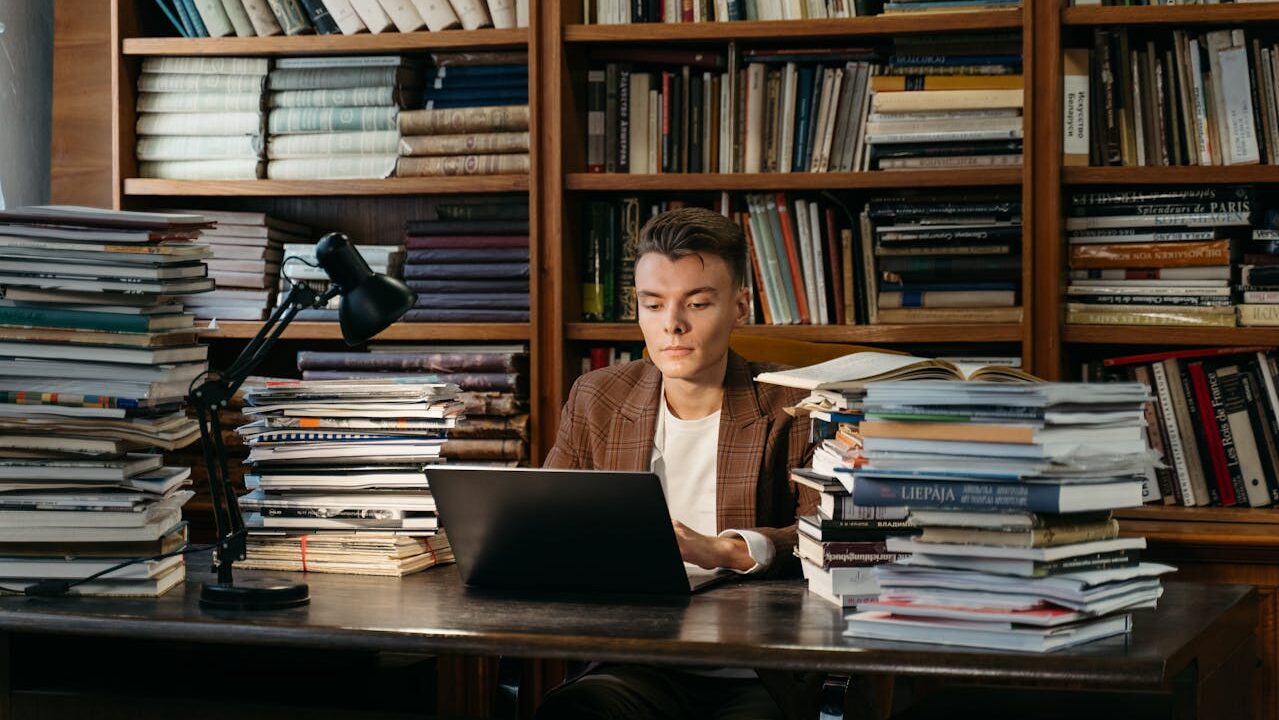
Common Errors in AI Detectors
AI busted tools are designed to identify content generated by artificial intelligence, but they are not infallible. Understanding the common errors in AI busted detectors can help you navigate their limitations effectively.
Easily Fooled Tricks
Many AI detectors are easily misled by simple tricks. Research from the University of Pennsylvania indicates that many open-source models for detecting AI content have “dangerously high” default false positive rates. Some of the most accurate detectors can fail dramatically when faced with minor technical challenges that would not confuse human evaluators.
Here are some common tricks that can fool AI detectors:
Trick | Description | Performance Drop |
---|---|---|
Adding Whitespace | Inserting spaces in unexpected places | ~30% |
Introducing Misspellings | Deliberately misspelling words | ~30% |
Selectively Paraphrasing | Changing phrases while keeping the meaning | ~30% |
Removing Grammatical Articles | Omitting “a,” “an,” and “the” | ~30% |
Using Homoglyphs | Replacing characters with visually similar ones | ~30% |
These tricks can significantly reduce the effectiveness of AI detection tools, leading to inaccurate assessments of content.
Generalization Challenges
AI detectors often struggle to generalize across different AI models. While many detectors excel at identifying content created by popular models like ChatGPT, they tend to perform poorly when analyzing text generated by lesser-known large language models (EdScoop). This inconsistency can lead to unreliable results, as the detectors may not recognize the unique patterns or characteristics of various AI-generated content.
Multiple studies have found that AI detectors are “neither accurate nor reliable,” producing a high number of false positives and false negatives. False positives can incorrectly flag human-written content as AI-generated, which can have serious repercussions, especially in academic settings. This creates an environment of distrust among users who rely on these tools for accurate assessments.
For more insights on the reliability of AI detectors, check out our article on how reliable are ai detectors?. If you’re curious about the accuracy of specific tools, you can explore what is the most accurate ai detection tool?.
Implications and Consequences
AI detectors are becoming increasingly common, but their reliability raises significant concerns. Understanding the implications of their errors is crucial for anyone using these tools.
False Positives Impact
One of the most pressing issues with AI detectors is the occurrence of false positives. These are instances where content created by humans is incorrectly flagged as being generated by AI. This can lead to serious repercussions, especially in academic settings. For example, students may face penalties that affect their academic records, creating an environment of distrust among educators and learners alike (University of San Diego School of Law).
The following table illustrates the potential impact of false positives in various scenarios:
Scenario | Potential Consequences |
---|---|
Academic Evaluations | Loss of grades, academic probation |
Job Applications | Disqualification from hiring processes |
Content Publishing | Rejection of articles or papers |
The critical flaw in AI detection tools is their unacceptably high rate of false positives, which can lead to devastating consequences for innocent individuals in high-stakes situations like academic evaluations or hiring processes (Christopher Penn).
Discriminatory Outcomes
False positives do not affect all individuals equally. Certain demographics, such as non-native English speakers and students with learning disabilities, are disproportionately impacted. These groups may face unfair punishment for being falsely accused of using AI-generated content, often without any recourse to overturn incorrect judgments.
This bias in AI detection can create a hostile environment, where individuals feel marginalized and unjustly treated. The following table summarizes the demographics most affected by false positives:
Demographic Group | Impact of False Positives |
---|---|
Non-native English Speakers | Increased likelihood of being flagged |
Students with Learning Disabilities | Unfair academic penalties |
Other Marginalized Groups | General distrust in AI detection systems |
Understanding these implications is essential for anyone involved in writing, marketing, or using AI detection tools. For more insights on the reliability of these systems, check out our article on how reliable are ai detectors?.