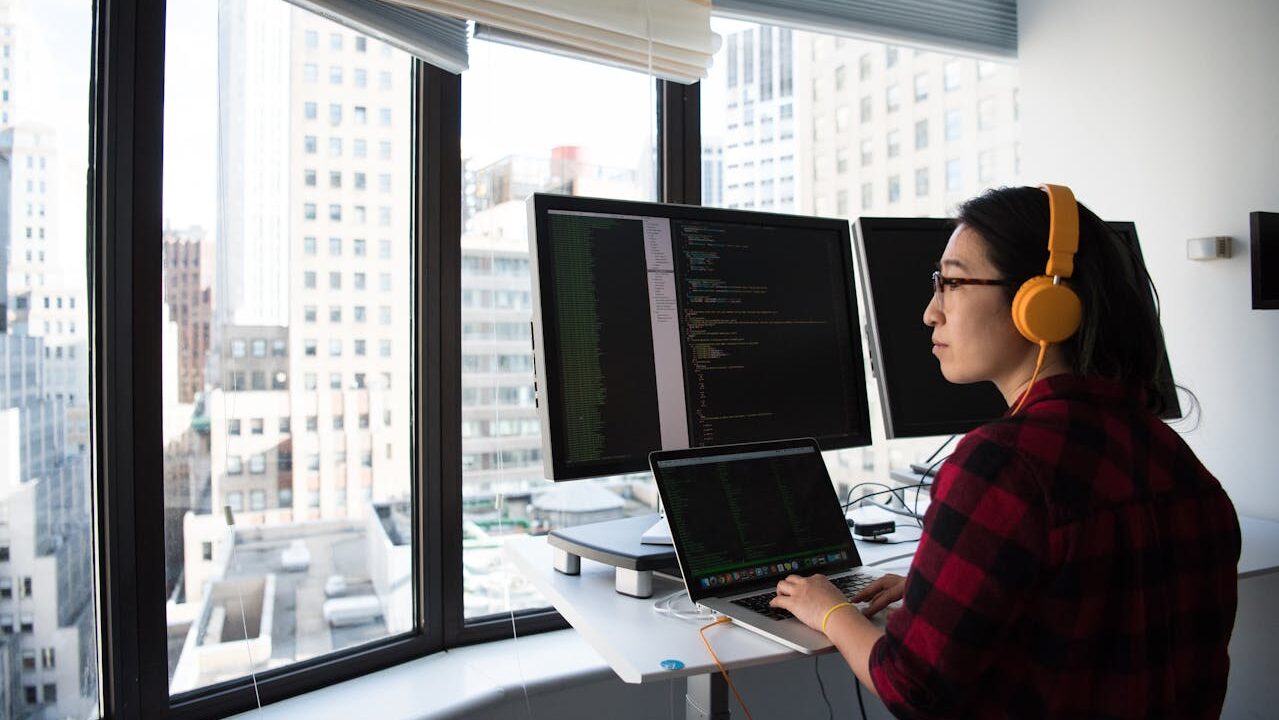
Understanding AI Failures
AI projects can be exciting and transformative, but they often come with their own set of challenges. AI busted initiatives highlight the need to understand why these projects fail and how to avoid the common pitfalls. By exploring the reasons behind AI failures, you can better navigate this complex landscape.
Common Causes of AI Failures
Studies show that up to 85% of AI projects fail, with poor data quality being the main culprit. AI systems are only as good as the data they are trained on. Using flawed, incomplete, or biased datasets leads to unreliable outputs (Univio). Here are some common causes of AI failures:
Cause of Failure | Description |
---|---|
Poor Data Quality | Inaccurate or biased data can lead to incorrect predictions. |
Lack of Transparency | When AI decision-making is opaque, it can exacerbate issues related to bias. |
Algorithmic Bias | AI systems may perpetuate existing biases, leading to unfair outcomes. |
Privacy Violations | Mismanagement of data can result in significant privacy breaches. |
Privacy violations and algorithmic bias account for more than four out of five failure cases. These issues not only affect the performance of AI systems but also raise ethical concerns.
Impact of AI Failures
The consequences of AI failures can be severe, especially in critical areas like healthcare. Errors in AI applications can lead to life-threatening misdiagnoses and lost opportunities for early intervention. The impact of AI failures can be categorized as follows:
Impact | Description |
---|---|
Reputational Damage | Companies may face backlash due to privacy violations or biased outcomes. |
Financial Loss | Failed AI projects can lead to wasted resources and lost revenue. |
Ethical Concerns | AI failures can raise questions about fairness and accountability. |
The fear of automation stems from AI’s failures to accurately replicate complex human judgment, perpetuating biases and errors.
AI busted scenarios often spark doubts about whether artificial intelligence can genuinely replace human roles on a large scale (Univio). Understanding these impacts can help you make informed decisions about AI implementation and management.
For more insights on what happens if a college board detects AI, check out our article on what happens if a college board detects ai?. If you’re curious about the specifics of AI detection, you can learn more about what does an ai detector look for? and the potential for false positives in AI detection.
Addressing AI Failures
AI projects can face significant challenges, but there are effective strategies to prevent failures and mitigate their consequences. Understanding these approaches can help you navigate the complexities of AI implementation.
Strategies to Prevent AI Failures
To reduce the risk of AI project failures, consider the following strategies:
- Ensure Data Quality: Studies indicate that up to 85% of AI projects fail, primarily due to poor data quality. AI systems rely heavily on the data they are trained on. Using flawed, incomplete, or biased datasets can lead to unreliable outputs. Focus on collecting high-quality, diverse data to improve the performance of your AI models.
- Train Your Team: Companies often overlook the importance of training staff on new technologies. If your team does not understand how an AI tool enhances their existing processes, they may resist adopting it. Invest in comprehensive training programs to help your staff see the value of AI in their work.
- Establish Clear Objectives: Define clear goals and expectations for your AI projects. This helps ensure that everyone involved understands the purpose and desired outcomes, reducing the likelihood of misalignment and confusion.
Strategy | Description |
---|---|
Ensure Data Quality | Focus on collecting high-quality, diverse datasets. |
Train Your Team | Provide comprehensive training on AI tools and their benefits. |
Establish Clear Objectives | Define clear goals and expectations for AI projects. |
Mitigating the Consequences
Even with preventive measures, AI failures can still occur. Here are ways to mitigate their consequences:
- Address Governance Issues: Privacy violations often stem from governance breakdowns rather than technical flaws. Organizations must tackle underlying governance problems to prevent reputational damage.
- Focus on Transparency: Lack of transparency in AI decision-making can exacerbate bias issues, particularly in fraud detection systems. This creates reputational risks and ethical concerns. Strive for transparency in your AI processes to build trust and accountability (Univio).
- Implement Safety Protocols: In critical areas like healthcare, errors can lead to life-threatening misdiagnoses. Establish safety protocols and regular audits to ensure that AI applications are functioning correctly and safely (United Nations University).
By adopting these strategies and focusing on governance and transparency, you can significantly reduce the risks associated with AI projects. For more insights on AI detection and its implications, check out our article on what happens if a college board detects AI?.