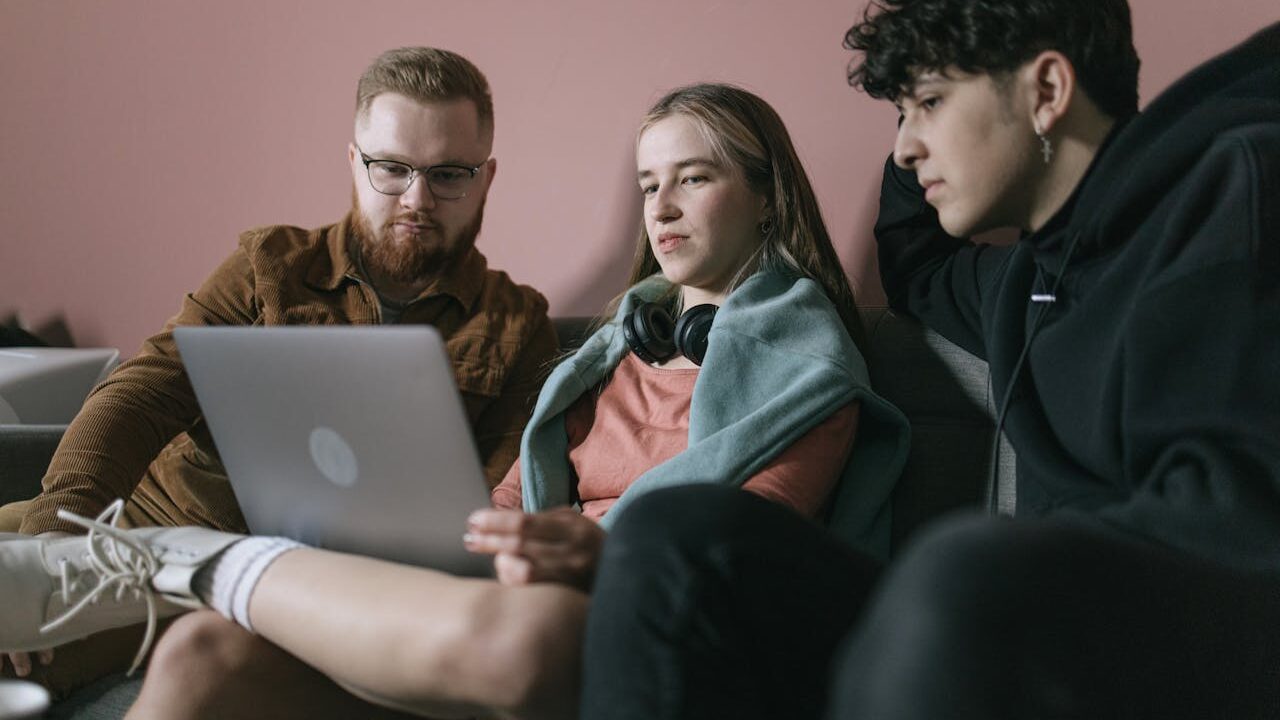
Understanding AI Detection Accuracy
As you explore the world of AI detection, it’s essential to grasp the factors that influence its accuracy and the implications of false positives. Understanding these elements can help you navigate the complexities of AI tools effectively.
Factors Influencing AI Accuracy
Several factors can impact the accuracy of AI detection systems. These include the quality of the training data, the algorithms used, and the specific context in which the AI operates. Here are some key elements to consider:
Factor | Description |
---|---|
Training Data Quality | High-quality, diverse datasets lead to better model performance. Poor data can result in inaccurate predictions. |
Algorithm Selection | Different algorithms have varying strengths and weaknesses. Choosing the right one is crucial for accurate detection. |
Contextual Relevance | AI systems must be tailored to the specific context in which they are applied to ensure accuracy. |
Using multiple AI detection tools with different algorithms can help cross-check results and enhance content reliability.
Impact of False Positives
False positives in AI detection can have serious consequences, particularly in academic settings where originality is vital. For instance, a false positive from Turnitin led to the banning of a specific feature, causing significant issues for educational institutions like Vanderbilt University.
False positives can unfairly target students with advanced writing styles, especially in technical fields. This can disproportionately affect high-performing students, international students, and technical writers, raising concerns about systemic bias.
Addressing false positives consumes valuable time and resources, diverting attention from essential tasks and affecting morale and productivity. This not only wastes institutional resources but also places an emotional burden on those involved.
Machine learning (ML) can significantly reduce false positives by building mathematical models from training data to identify normal patterns and distinguish them from anomalies. In one case study, ML achieved a 60% reduction in false positives compared to traditional rule-based systems (Softjourn).
Understanding these factors and impacts is crucial as you consider how to avoid false positive AI detection in your work. For more insights, check out our article on are al detectors accurate in 2025?.
Strategies to Improve AI Detection
To enhance the accuracy of AI detection and minimize false positives, you can implement several effective strategies. Two key approaches include utilizing regularization techniques and incorporating diverse training datasets.
Regularization Techniques
Regularization is a powerful method to improve the performance of AI models. It works by penalizing the model for making extreme predictions, which helps to prevent overfitting and reduces the likelihood of incorrect outcomes. By limiting the number of possible predictions, you can effectively reduce AI hallucinations, which are instances where the AI generates inaccurate or misleading information.
Here’s a summary of how regularization can help:
Benefit | Description |
---|---|
Reduces Overfitting | Prevents the model from becoming too tailored to the training data. |
Enhances Generalization | Improves the model’s ability to make accurate predictions on new, unseen data. |
Lowers False Positives | Helps in minimizing incorrect classifications by maintaining a balanced prediction approach. |
Incorporating regularization techniques into your AI model can significantly enhance its reliability. For more information on AI accuracy, check out our article on are al detectors accurate in 2025?.
Diverse Training Datasets
Another effective strategy to reduce false positives in AI detection is to use diverse training datasets. By including a wide array of writing styles and genres, you can help the AI model learn to recognize various patterns and contexts. This broad exposure allows the model to better differentiate between genuine content and AI-generated text, ultimately improving its accuracy.
Research shows that platforms that updated their algorithms and diversified their training datasets managed to cut sentence-level false positives by up to 75% (Detecting AI).
Here’s a breakdown of the advantages of diverse training datasets:
Advantage | Description |
---|---|
Improved Accuracy | A wider range of examples helps the model learn more effectively. |
Reduced False Positives | Better differentiation between human and AI-generated content. |
Enhanced Adaptability | The model can adjust to various writing styles and contexts. |
By focusing on these strategies, you can significantly enhance the performance of AI detection systems. For further insights into AI detection accuracy, consider exploring our article on can ai be 100 accurate?.