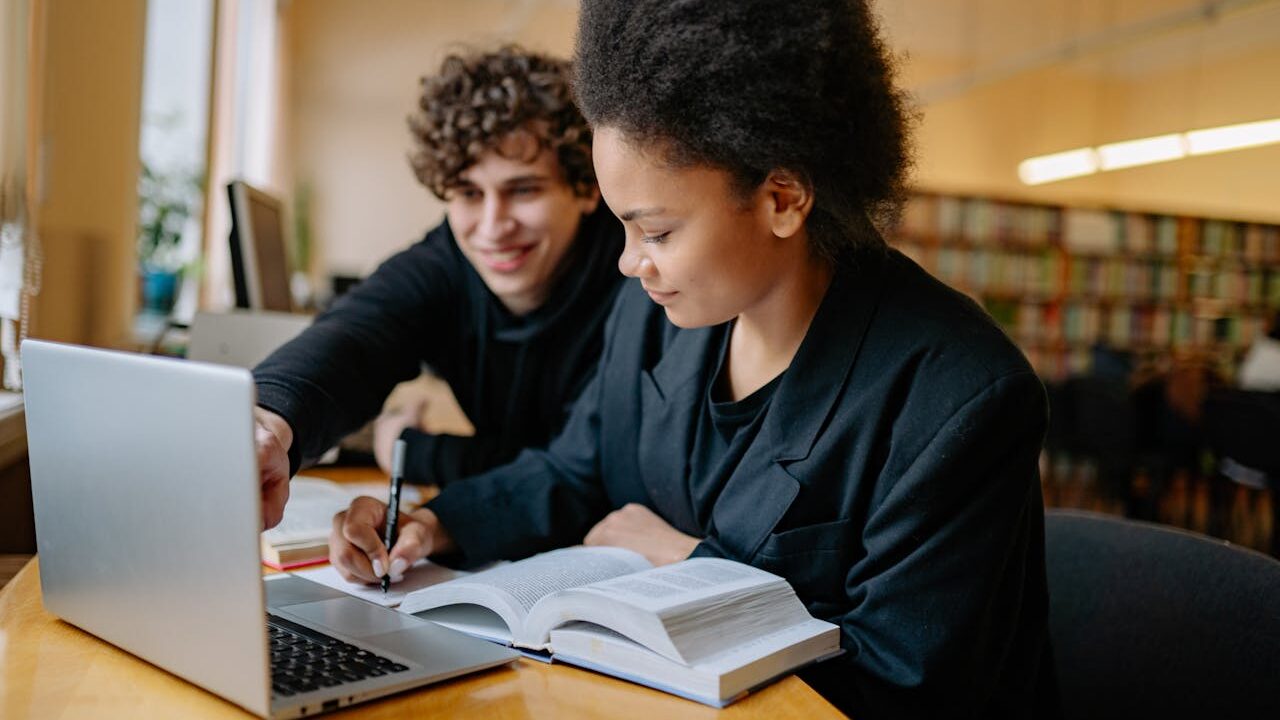
Understanding AI Detectors
AI busted detectors are essential tools in today’s digital landscape, especially for writers and marketers. They help identify whether content has been generated by artificial intelligence. Understanding their role and the importance of accurate detection can enhance your content creation and evaluation processes.
Role of AI Detectors
AI busted tools serve a critical function by analyzing various forms of content, including text, images, video, audio, and code. They utilize complex algorithms to examine syntax, word usage, language patterns, and other clues to determine if AI was involved in content creation (VidCruiter).
These tools can provide a confidence score, indicating the likelihood that the content is AI-generated. Different detectors may present results in various formats, such as a percentage of AI-generated text or a confidence level regarding AI involvement. This information can be invaluable for ensuring the authenticity of content, especially in fields like marketing and academia.
Detector Type | Content Analyzed | Output Format |
---|---|---|
Text Detectors | Text | Confidence Score (%) |
Image Detectors | Images | AI Involvement Level |
Audio Detectors | Audio | Detection Percentage |
Importance of Accurate Detection
Accurate detection is crucial for several reasons. First, it helps maintain the integrity of content, especially in high-stakes scenarios like academic evaluations or hiring processes. Relying solely on AI detectors for decision-making can be risky, as these tools have limitations in accuracy. Human oversight is essential to ensure that decisions are well-informed and fair.
Moreover, understanding the potential errors in AI detection can help you navigate the challenges associated with these tools. For instance, AI-generated content is designed to closely mimic human writing, making it difficult for detectors to consistently identify it. This is why it’s important to be aware of the errors in AI detectors and to consider using multiple tools for a more comprehensive evaluation.
For more insights on the reliability of these tools, check out our article on how reliable are ai detectors?. If you’re curious about what constitutes a good score for AI detection, visit what is a good score for ai detection?. Additionally, you can explore whether AI can be detected in papers by reading can ai be detected in papers?.
Challenges in AI Detection
AI detection tools face several challenges that can impact their effectiveness. Understanding these challenges is crucial for anyone interested in the accuracy of these systems.
Human Biases in AI Systems
Human biases can infiltrate artificial intelligence systems, leading to potentially harmful outcomes. This issue has gained attention as many companies implement AI across various operations. Bias can occur at every stage of AI model development, from problem definition to post-deployment monitoring. Efforts to detect and mitigate bias should be made throughout the entire process (Harvard Business Review).
Bias in AI can be intentional or unintentional, introducing systematic errors into the detection process. For instance, if an AI detector is trained on biased data, it may produce skewed results, misclassifying human-written content as AI-generated or vice versa. This can lead to a lack of trust in AI detection tools and their outputs.
Stage of AI Development | Potential Bias Issues |
---|---|
Problem Definition | Misalignment of objectives |
Data Collection | Non-representative samples |
Model Training | Overfitting to biased data |
Post-Deployment | Lack of ongoing monitoring |
Efforts to mitigate biases in AI models are often piecemeal, typically conducted only during model development and validation (NCBI). This lack of a unified approach makes it difficult to ensure that AI detectors are free from bias.
Factors Affecting Detection Accuracy
Several factors can influence the accuracy of AI detection tools. AI detectors rely on complex algorithms that analyze text patterns, sentence structure, word repetition, and the complexity of ideas presented. However, AI-generated content is designed to closely mimic human writing, making it challenging to detect consistently (VidCruiter).
Some key factors affecting detection accuracy include:
- Quality of Training Data: If the training data is not diverse or representative, the AI detector may struggle to generalize effectively.
- Algorithm Complexity: More complex algorithms may improve detection but can also introduce new errors if not properly calibrated.
- Evolving AI Writing Styles: As AI writing tools become more sophisticated, they may produce content that is increasingly difficult to distinguish from human writing.
Factor | Impact on Detection Accuracy |
---|---|
Quality of Training Data | High-quality, diverse data improves accuracy |
Algorithm Complexity | Complex algorithms can enhance detection but may also introduce errors |
Evolving AI Writing Styles | More sophisticated AI writing makes detection harder |
Understanding these challenges can help you navigate the landscape of AI detection tools more effectively. For more insights on the limitations of these systems, check out our article on what are the errors in ai detectors?.