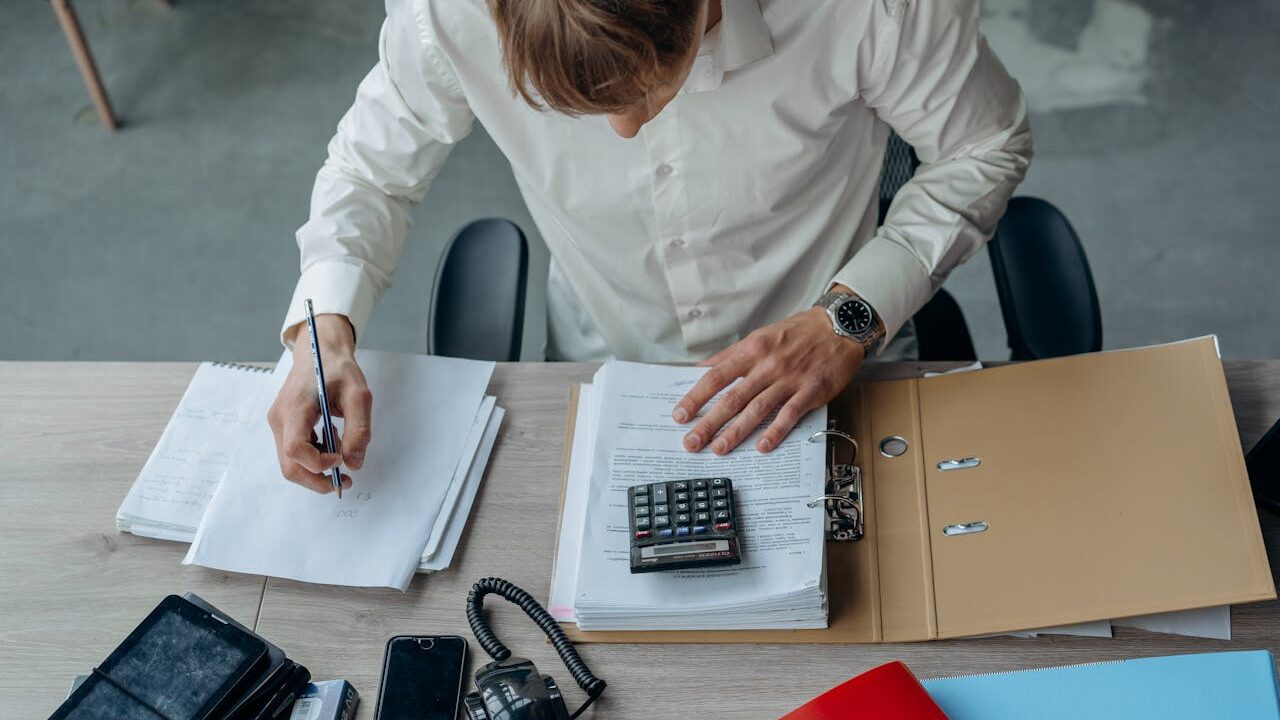
Challenges in AI Detection
AI detection tools are designed to identify content generated by artificial intelligence, but they come with their own set of challenges. The emergence of AI busted cases highlights the need for better understanding these issues. This knowledge is crucial for anyone involved in writing, marketing, or using AI technologies.
False Positives in AI Detection
A significant problem with AI detection is the occurrence of false positives. This happens when a tool incorrectly identifies human-written text as AI-generated. For instance, Turnitin’s AI writing detection aims for a high accuracy rate, maintaining a false positive rate of less than 1% to avoid falsely accusing students of misconduct (Turnitin). However, in a Bloomberg test, false positive rates ranged from 1-2% when analyzing a sample of 500 essays, which could lead to millions of essays being wrongly flagged (Center for Innovative Teaching and Learning).
The consequences of false positives can be severe. Students may face academic penalties, loss of scholarships, and damage to their future opportunities. This issue raises concerns about the reliability of AI detection tools, especially in educational settings where maintaining academic integrity is paramount.
False Positive Rate | Potential Impact |
---|---|
Less than 1% | Minimal impact on students |
1-2% | Millions of falsely flagged essays, serious academic consequences |
Lack of Transparency in AI Models
Another challenge in AI detection is the lack of transparency in the models used. Many AI detection tools rely on complex algorithms to differentiate between human and AI-generated text. However, the accuracy of these tools can vary significantly based on the complexity of the text and the methods used to disguise AI-generated content (Center for Innovative Teaching and Learning).
This lack of transparency can lead to distrust among users, particularly in educational environments. If students and educators do not understand how these tools work, they may question the fairness of the assessments. Additionally, biases in AI models can disproportionately affect certain groups, such as non-native English speakers and neurodiverse students, further complicating the issue. By recognizing AI busted scenarios, it becomes evident why transparency is so vital.
For more insights into the reliability of AI detection tools, you can explore our article on can AI detection be wrong?. Understanding these challenges is essential for navigating the evolving landscape of AI technologies and their implications for writing and education.
Ethical Considerations in AI Detection
As you explore the landscape of AI detection, it’s essential to consider the ethical implications that come with it. Two significant concerns are bias in AI models and privacy issues related to AI detection.
Bias in AI Models
Bias in AI models can lead to unfair outcomes and reinforce existing stereotypes. When AI systems are trained on data that reflects societal biases, they can inadvertently perpetuate these biases in their predictions and decisions. This is particularly concerning in areas like hiring, law enforcement, and lending, where biased AI can have serious consequences for individuals and communities.
To address bias, it’s crucial to implement fairness measures in AI development. This includes diversifying training data, regularly auditing AI systems for bias, and ensuring that AI models are transparent and accountable. Companies must align their AI systems with societal expectations and foster a culture of responsibility to mitigate these risks (Lumenalta).
Type of Bias | Description | Example |
---|---|---|
Data Bias | Arises from unrepresentative training data | AI hiring tool favoring one demographic |
Algorithmic Bias | Results from flawed algorithms | Predictive policing tools targeting specific neighborhoods |
Human Bias | Reflects biases of developers | AI systems that mirror developer prejudices |
Privacy Concerns in AI Detection
Privacy concerns are another critical aspect of AI detection. As AI systems often require vast amounts of data to function effectively, the collection and storage of personal information can lead to significant privacy risks. Users may not be fully aware of how their data is being used, leading to a lack of trust in AI technologies.
To protect privacy, companies must prioritize data protection and transparency. Implementing robust regulatory frameworks can help ensure that AI practices align with ethical standards and respect user privacy (Lumenalta). It’s essential to communicate clearly with users about data usage and to provide options for data control.
Privacy Concern | Description | Mitigation Strategy |
---|---|---|
Data Collection | Gathering personal information without consent | Obtain explicit user consent |
Data Storage | Storing sensitive data insecurely | Use encryption and secure storage solutions |
Data Usage | Using data for unintended purposes | Clearly define data usage policies |
By addressing these ethical considerations, you can better understand the complexities surrounding AI detection. For more insights on the challenges of AI detection, check out our article on what are the problems with ai detection?. If you’re curious about the accuracy of AI detection, you might want to read about can AI detection be wrong?.