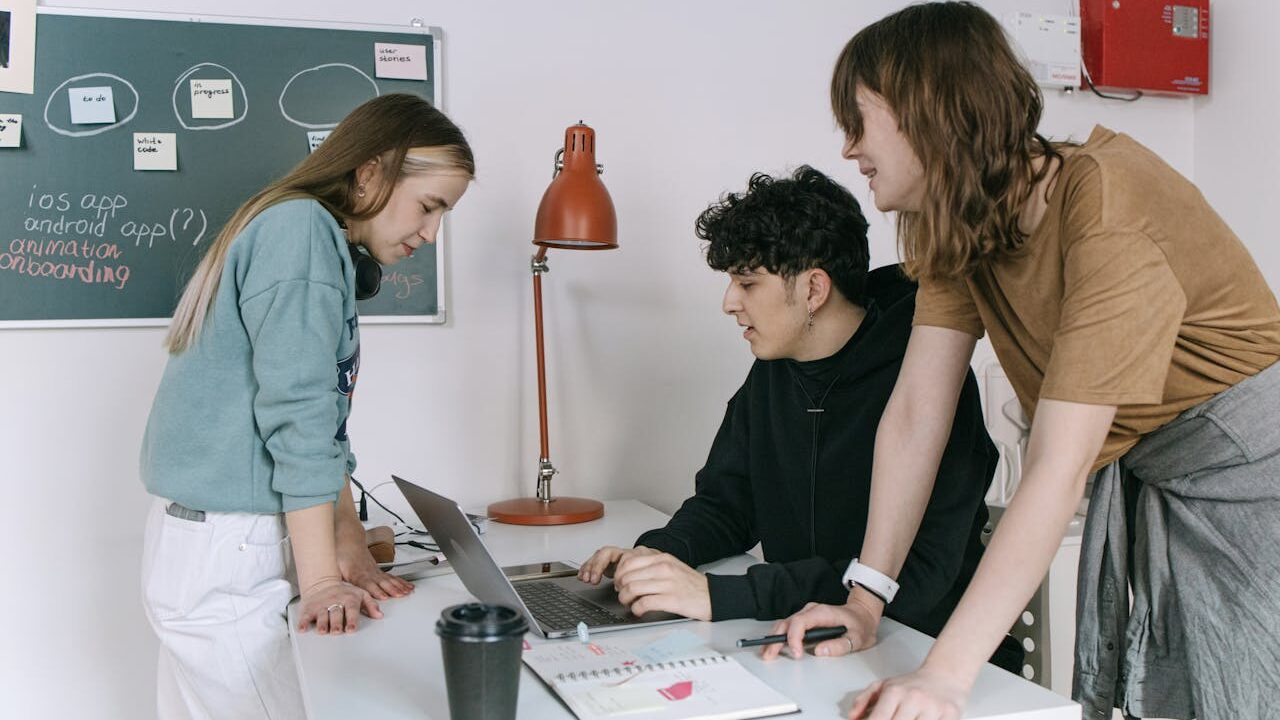
Accuracy of AI Detectors
As you explore the world of AI detectors, understanding their accuracy is crucial. In 2025, the effectiveness of these tools is influenced by various factors, including the impact of generative AI tools and the risks associated with AI hallucinations.
Impact of Generative AI Tools
Generative AI tools, such as Stable Diffusion, have been shown to amplify biases, including gender and racial stereotypes, in the content they produce. In a study involving over 5,000 images, these biases were highlighted, raising concerns about the reliability of AI-generated content (Bloomberg Technology + Equality). This bias can affect the accuracy of AI detectors, as they may struggle to differentiate between biased content and authentic material.
Type of Bias | Example |
---|---|
Gender Bias | Amplification of stereotypes in images |
Racial Bias | Misrepresentation of racial groups in outputs |
As some users attempt to navigate the detection landscape, they may fear being ai busted due to these biases in AI-generated content.
The presence of these biases can lead to inaccuracies in AI detection systems, making it essential for users to remain vigilant when relying on these tools.
Risks of AI Hallucinations
AI hallucinations refer to instances where AI tools, like ChatGPT, generate fabricated data that appears authentic. This phenomenon has been documented in various scenarios, including a legal case where ChatGPT produced misleading citations and quotes that did not exist. Such inaccuracies can significantly impact the reliability of AI detectors.
AI systems, including ChatGPT, Copilot, and Gemini, have been known to provide users with misleading information, leading to the coining of the term “hallucinations” for these inaccuracies. This raises questions about the overall accuracy of AI detectors in identifying genuine content versus AI-generated fabrications.
Type of Hallucination | Description |
---|---|
Fabricated Data | AI generates false information that seems real |
Misleading Outputs | AI provides incorrect citations or quotes |
As you consider the question, can AI be 100% accurate?, it’s important to recognize the limitations of AI detectors in light of these challenges. Understanding the impact of generative AI tools and the risks of hallucinations can help you navigate the complexities of AI detection more effectively. For more insights, check out our article on are al detectors accurate in 2025?.
Addressing AI Accuracy Challenges
As you explore the question of whether AI can be 100% accurate, it’s essential to consider the challenges that affect AI accuracy. Two significant factors are bias in AI systems and the importance of human oversight.
Bias in AI Systems
AI systems can amplify biases present in historical data, leading to discriminatory results in various fields such as lending, hiring, and criminal justice. This perpetuates existing social inequalities and can result in a less diverse and inclusive workforce.
When AI is trained on biased data, it produces biased outcomes, regardless of how accurate its predictions may seem. For instance, an AI used in criminal justice that relies on historical crime data may disproportionately impact specific communities, reflecting and perpetuating societal biases rather than presenting an objective truth (United Nations University).
The table below illustrates some areas where bias can manifest in AI systems:
Area | Potential Bias Impact |
---|---|
Lending | Systematic disparate treatment of marginalized consumers |
Hiring | Lack of diversity in candidate selection |
Criminal Justice | Disproportionate effects on specific communities |
AI-driven decisions in lending can replicate past failings in the banking industry, leading to practices like redlining if not carefully monitored.
Importance of Human Oversight
Keeping a human in the loop is a widely recommended approach to overseeing AI systems. This helps maintain trust and mitigate risks associated with AI-generated content. However, many individuals tend to rely heavily on AI outputs, even when aware of potential errors. This reliance can lead to significant consequences, affecting investments, livelihoods, and decision-making processes.
Currently, companies that develop or utilize AI systems largely self-regulate, depending on existing laws and market forces to guide their practices. There is little consensus on how AI should be regulated, raising concerns about the ability of government regulators to keep pace with rapid technological advancements.
To ensure AI systems are as accurate as possible, it is crucial to implement robust human oversight and continuously evaluate the data used for training. For more insights on AI detection accuracy, check out our article on are al detectors accurate in 2025?.