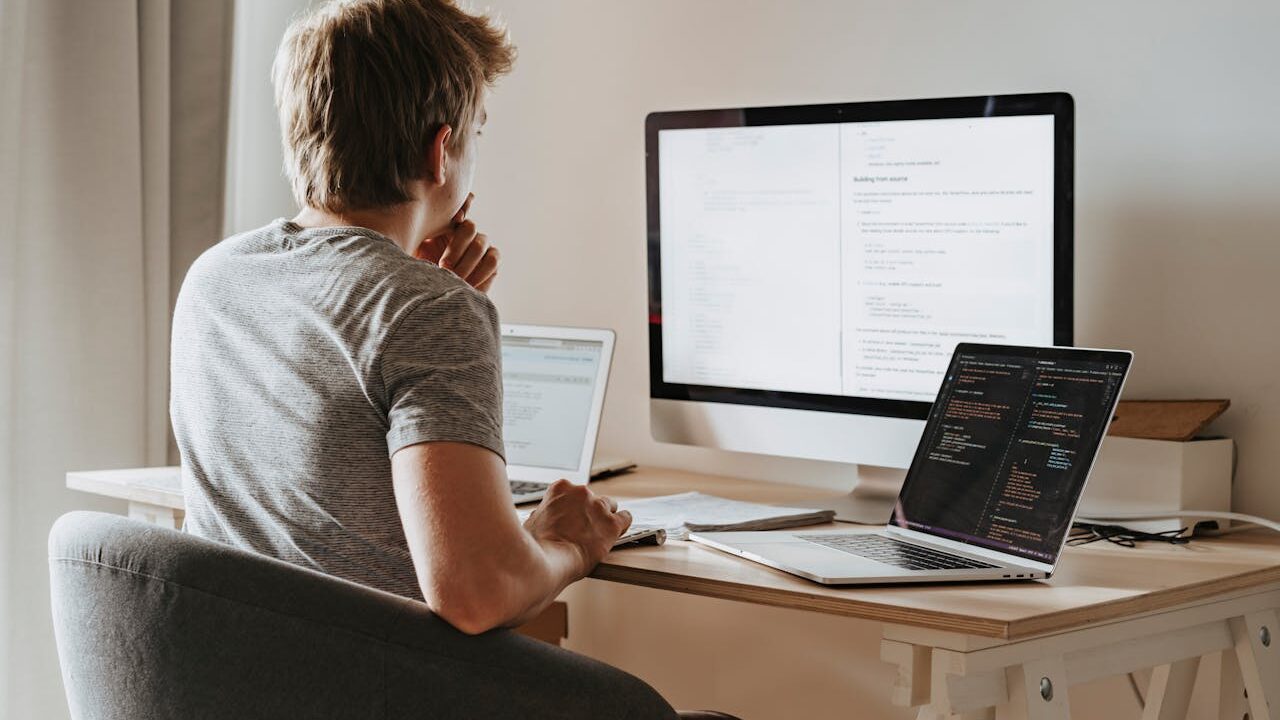
Understanding AI Detection Tools
As AI technology continues to evolve, so do the tools designed to detect AI-generated content. Understanding how these detection tools work and their limitations is essential for anyone involved in writing, marketing, or AI development. AI busted scenarios highlight the challenges and inconsistencies in the current generation of detection tools.
Inconsistencies in AI Detection
AI detection tools are not infallible. A study published in the International Journal for Educational Integrity found that when these tools were applied to human-written responses, they exhibited inconsistencies. This led to false positives and uncertain classifications, indicating a need for further development and refinement of these tools.
The OpenAI classifier, for example, accurately identified only 26% of AI-written text as “likely AI-generated.” However, it incorrectly labeled 9% of human-written text as AI-generated, showcasing a significant false positive rate. This inconsistency can create confusion and mistrust in the detection process.
Detection Tool | AI Detection Accuracy | False Positive Rate |
---|---|---|
OpenAI Classifier | 26% | 9% |
Turnitin.com | N/A | 1% |
False Positives in AI Detection
False positives occur when an AI detection tool mistakenly identifies human-written content as AI-generated. This can have serious implications, especially in academic settings. For instance, if a college board detects AI in a student’s work, it could lead to disciplinary actions based on incorrect assumptions.
Turnitin.com claims a false positive rate of just 1% for post-secondary writing, which is among the lowest rates reported. However, even a small percentage can lead to significant issues if it affects a large number of submissions.
The implications of false positives extend beyond academia. In cybersecurity, for example, false positives can disrupt regular operations and consume valuable resources, as noted in LinkedIn. AI busted examples of false positives highlight the importance of addressing these inaccuracies to maintain trust and efficiency.
For more insights on what happens if a college board detects AI, check out our article on what happens if a college board detects ai?. Understanding the limitations of AI detection tools can help you navigate the complexities of AI-generated content and its implications.
Implications and Solutions
Impact of False Positives
When AI detection tools incorrectly label human-written content as AI-generated, it can lead to significant consequences. This phenomenon, known as a false positive, can create confusion and mistrust among writers, educators, and institutions. For instance, if a college board detects AI in a student’s work, it may result in academic penalties or even expulsion, impacting the student’s future opportunities.
The study found that the OpenAI classifier inaccurately identified 9% of human-written text as AI-generated, highlighting the potential risks involved (International Journal for Educational Integrity). This inconsistency can undermine the credibility of AI detection tools and lead to a lack of confidence in their results.
Consequence of False Positives | Description |
---|---|
Academic Penalties | Students may face disciplinary actions for suspected cheating. |
Mistrust in Tools | Writers and educators may doubt the reliability of AI detection tools. |
Impact on Reputation | A false positive can harm a student’s academic record and future prospects. |
Strategies to Address False Positives
To mitigate the risks associated with false positives, several strategies can be implemented. These include improving the accuracy of AI detection tools, providing clear guidelines for users, and fostering open communication between institutions and students.
- Enhancing AI Detection Tools: Continuous development and refinement of AI detection algorithms are essential. The study indicated that while some tools, like OpenAI, demonstrated high sensitivity, they struggled with specificity (International Journal for Educational Integrity). Investing in better technology can help reduce false positives.
- Clear Guidelines: Institutions should establish clear policies regarding the use of AI detection tools. This includes outlining the consequences of false positives and providing students with information on how to avoid being misclassified.
- Open Communication: Encouraging dialogue between students and educators can help address concerns related to AI detection. If a student believes their work has been incorrectly flagged, they should have a clear process for appeal and review.
- Education on AI Tools: Providing training for both students and educators on how AI detection tools work can foster understanding and reduce anxiety surrounding their use. This can include discussions on what AI detectors look for and how to create content that minimizes the risk of misclassification.
By implementing these strategies, the impact of false positives can be significantly reduced, leading to a more trustworthy and effective use of AI detection tools in academic settings. For more insights on the reliability of AI detection, check out our article on what percentage of AI fails?.